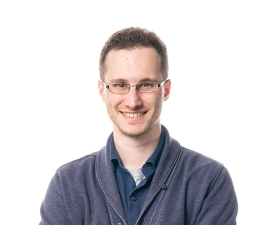
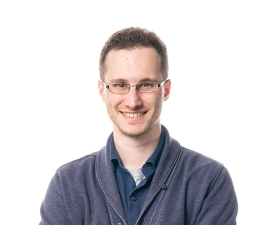
Research Expertise and Interest
artificial intelligence, intelligent systems and robotics
Research Description
Sergey Levine received a BS and MS in Computer Science from Stanford University in 2009, and a Ph.D. in Computer Science from Stanford University in 2014. He joined the faculty of the Department of Electrical Engineering and Computer Sciences at UC Berkeley in fall 2016. His work focuses on machine learning for decision making and control, with an emphasis on deep learning and reinforcement learning algorithms. Applications of his work include autonomous robots and vehicles, as well as computer vision and graphics. His research includes developing algorithms for end-to-end training of deep neural network policies that combine perception and control, scalable algorithms for inverse reinforcement learning, deep reinforcement learning algorithms, and more.
In the News
A Path to Resourceful Autonomous Agents
Learning to learn
Seven early-career faculty win Sloan Research Fellowships
Featured in the Media
How 34 labs are teaming up to tackle robotic learning.