With AI, machines become expert at reading brain scans
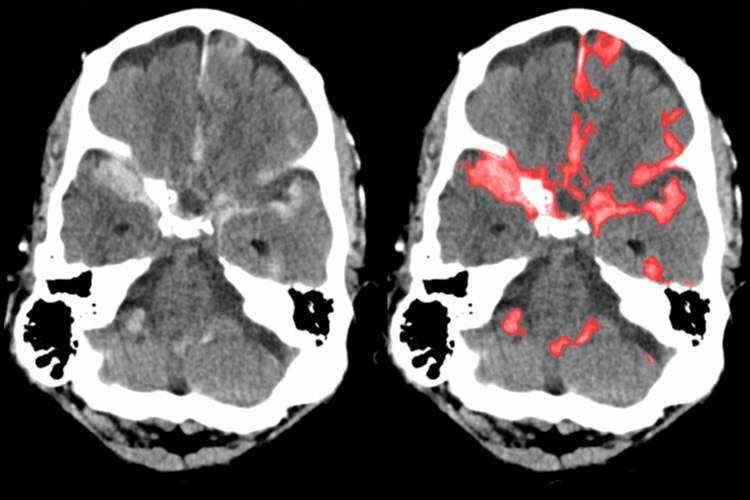
A computer algorithm developed by scientists at the University of California, San Francisco (UCSF), and UC Berkeley bested two out of four expert radiologists at finding tiny brain hemorrhages in head scans — an advance that one day may help doctors treat patients with traumatic brain injuries, strokes and aneurysms.
Radiologists typically look at thousands of brain images each day, searching for tiny abnormalities that can signal life-threatening emergencies. A single, three-dimensional, computed tomography scan can produce a stack of 30 or more images, each of which must be reviewed by a radiologist.
The researchers created their algorithm to see if artificial intelligence could more efficiently and accurately pick out images with significant abnormalities to help radiologists focus on the most important images and examine them more closely.
“We wanted something that was practical, and for this technology to be useful clinically, the accuracy level needs to be close to perfect,” said study co-author Esther Yuh, an associate professor of radiology at UCSF. “The performance bar is high for this application, due to the potential consequences of a missed abnormality, and people won’t tolerate less than human performance or accuracy.”
The algorithm that the team developed took just one second to determine whether an entire head scan contained any signs of hemorrhage. It also traced the detailed outlines of the abnormalities within the brain’s three-dimensional structure, with acceptable levels of false positives, minimizing the amount of time that physicians would need to spend reviewing its results. Some spots can be on the order of 100 pixels in size, and even expert radiologists sometimes miss them, with potentially grave consequences.
“Given the large number of people who suffer from traumatic brain injury every day and are rushed to the emergency department, this has very big clinical importance,” said co-author Jitendra Malik, the Arthur J. Chick Professor of Electrical Engineering and Computer Sciences at UC Berkeley.
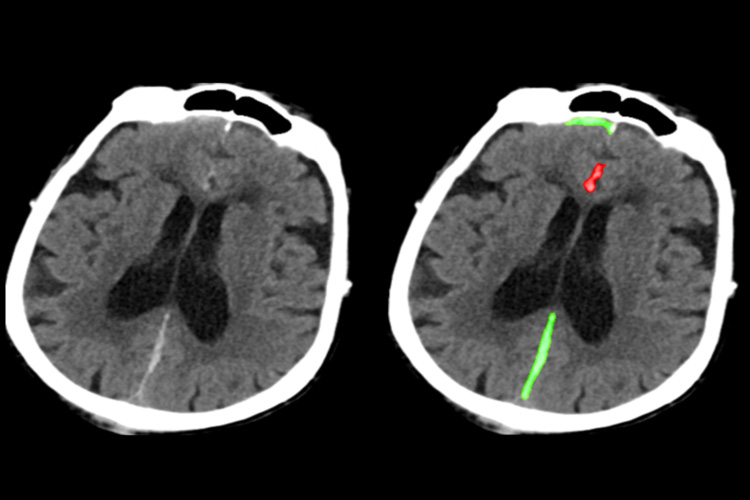
The algorithm found some small abnormalities that the experts missed. It also noted their location within the brain and classified them according to subtype, information that physicians need to determine the best treatment.
According to Malik, the key was choosing which data to feed into the model. The new study made use of a type of deep learning known as a fully convolutional neural network, or FCN, which trains algorithms on a relatively small number of images, in this case 4,396 CT exams. But the training images used by the researchers were packed with information, because each small abnormality was manually delineated at the pixel level. The richness of this data — along with other steps that prevented the model from misinterpreting random variations, or “noise,” as meaningful — created an extremely accurate algorithm.
The authors are now applying the algorithm to CT scans from trauma centers across the country that are enrolled in a research study led by UCSF’s Geoffrey Manley, a professor and vice chair of neurosurgery.
The study, which was co-authored by Weicheng Kuo and Christian Hӓne of UC Berkeley and Pratik Mukherjee of UCSF, appeared online today in the journal Proceedings of the National Academy of Sciences.