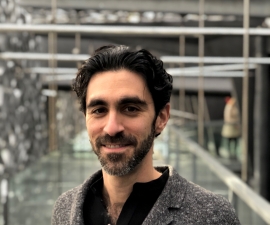
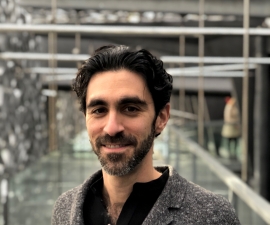
Research Expertise and Interest
machine learning, and medicine, health policy
Research Description
Ziad Obermeyer works at the intersection of machine learning and health. His research focuses on how machine learning can help doctors make better decisions (like whom to test for heart attack), and help researchers make new discoveries—by ‘seeing’ the world the way algorithms do (like finding new causes of pain that doctors miss, or linking individual body temperature set points to health outcomes). He has also shown how widely-used algorithms affecting millions of patients automate and scale up racial bias. That work has impacted how many organizations build and use algorithms, and how lawmakers and regulators hold AI accountable.
He is one of TIME Magazine's 100 most influential people in AI, a Chan–Zuckerberg Biohub Investigator, a Research Associate at the National Bureau of Economic Research, and was named an Emerging Leader by the National Academy of Medicine. Previously, he was Assistant Professor at Harvard Medical School, and continues to practice emergency medicine in underserved communities.