Satellite images, phone data help guide pandemic aid in at-risk developing countries
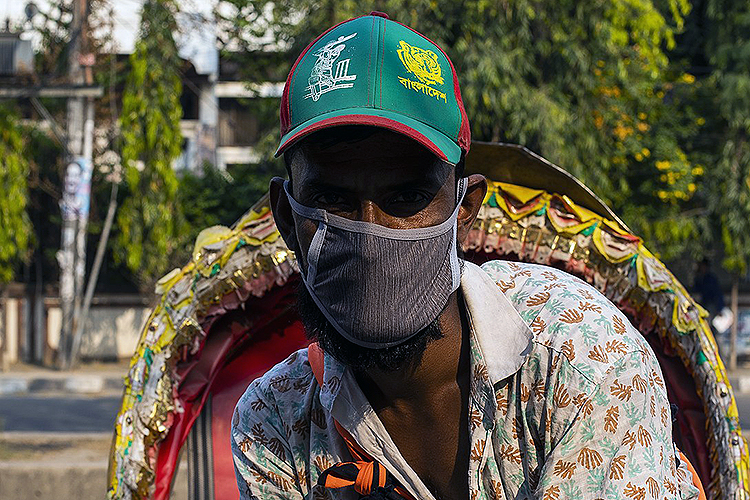
For some of the poorest countries on Earth, the COVID-19 pandemic has posed a life-or-death quandary: If people continue to work, the virus might spread unchecked. But if they have to stay at home to limit the contagion, hunger and malnutrition could soar.
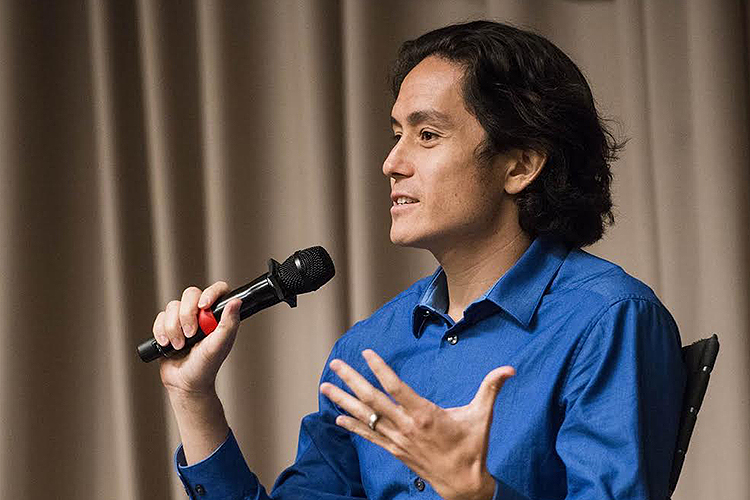
In the West African nation of Togo, policymakers decided that modest support payments to the neediest people are part of the solution — and they turned for help to the Center for Effective Global Action (CEGA) at UC Berkeley. The partnership has explored how artificial intelligence — driven by big data and machine learning, satellite images and phone records — can help to pinpoint the areas where needs are most urgent.
In recent weeks, CEGA faculty Co-director Joshua Blumenstock has worked with policymakers in Togo and Nigeria in Africa and in Bangladesh in South Asia to focus the power of advanced technology on pandemic relief. Blumenstock detailed these projects in an article published by Nature on May 14.
Technology is not an answer by itself, Blumenstock said in a recent interview, but it can be a powerful tool for governments or agencies with a humanitarian mission. And, he said, such work exemplifies the ideals and on-the-ground potential of Berkeley’s new Division of Computing, Data Science, and Society.
In addition to his post at CEGA, Blumenstock is an assistant professor at the Berkeley School of Information and director of the Data-Intensive Development Lab. CEGA works with partners around the world to alleviate poverty and advance social change through research and innovation.
[This interview has been lightly edited for length and clarity.]
Berkeley News: Let’s start with the basics: What is machine learning, and how does it work?
Joshua Blumenstock: Machine learning is a branch of artificial intelligence that’s generally thought of as a method for finding patterns in data. It’s a way to design a system that can learn and improve itself without being explicitly programmed. It’s a fundamental technology that underlies most of the online services we use today, things like Google and YouTube and Netflix and Spotify – but also offline decisions like hiring and firing and lending and medical treatment. It’s also used to transcribe the classes I teach.
How are governments in the developing world using these tools now, during the COVID-19 pandemic?
There’s an acute humanitarian crisis in developing countries that’s being driven by the coronavirus pandemic, and it’s projected to get worse. Countries all over the world are instituting the same lockdown policies that we see here in the states — stay-at-home orders that stop people from working. The key difference is that, in a lot of developing countries, very large portions of the population don’t have much in the way of savings or access to social protections.
A recent report by the World Food Program projected over a quarter of a billion people are likely to be going hungry in developing countries by the end of the year. And a lot of this is due to the economic consequences of COVID-19.
Policymakers who are trying to address this hunger pandemic need to know which people need aid the most. There aren’t enough resources to do something like a universal basic income, where you give support to everyone. And so, they have this really difficult problem: ‘Who should we give these limited resources to?’
But they can’t really answer that question using traditional data. For example, in a place like Nigeria, a lot of income is informal. Almost no one files taxes. The government can’t see who’s poor and who’s rich just by looking at income tax returns.
That’s where these new tools come in. What we’re doing is using data from satellites and mobile phone networks to try and identify specific regions or specific mobile subscribers that are very likely to be in need. Machine learning provides a way for processing and making sense of these big, non-traditional data sources.
What would satellite images or mobile phone usage patterns tell us about who’s in need?
Wealthy regions just look different than poor regions. The roofs are made out of different material. The streets are of different quality. The farm plots are different sizes. And all of this is very informative about which regions are wealthy and which are poor. A human could look at an image and maybe figure that out, but you need algorithms to do this at the scale of an entire country of 195 million people.
With phone data, it’s a very similar idea. Wealthy people use their phones differently than poor people. Wealthy people tend to make longer calls. They tend to make international calls. They tend to add credit to their phone in larger denominations. We’ve shown in papers over the last several years that machine learning algorithms can pick up on these patterns and use them to develop accurate predictions of the socioeconomic status of individual mobile phone owners.
But is there the capacity to identify specific communities that have been hard hit by the pandemic?
We’re working on that right now. Some of my earlier work shows that you can detect when people have negative, as well as positive, economic shocks based on how they’re using their phone. Based on satellite imagery, you can see over time how road quality changes. But we’re still trying to understand how accurately you can assess whether a household has been impacted by COVID-19. This requires new data collection.
And this is an important point: The new machine learning models are only as good as the data that are used to train them. They’re not meant to replace traditional forms of measurement, like survey- based data collection, but rather to complement them. So, what we’re doing in Togo and Bangladesh, for instance, is conducting rapid-fire phone surveys to ask people about how COVID-19 it is affecting them. Then, we can use that data to calibrate the predictive models.
You’re working with Togo, Nigeria and Bangladesh. What are they hoping to achieve with these new tools?
Their primary objective is really just to manage the humanitarian crisis that’s unfolding. The policymakers are extremely hard-working — they’re staying up to talk to us at 3 a.m. in Togo because they want to be sure that their fellow countrymen don’t starve.
In Togo, they have an existing social protection program that’s based on traditional government registry data. It’s a very impressive program, but they’re worried that maybe there are people slipping through the cracks. And so, they say: ‘Can the satellite data and the phone data help us identify those people?’
What are the challenges those governments face?
One critical aspect of this approach is to figure out how to respond to the crisis in a way that solves the immediate objective, but doesn’t compromise on ethical or privacy standards — which might have longer-term consequences.
The United States is a prime example of where national crises have led to lowered restrictions on personal privacy. I’m thinking specifically of the USA PATRIOT Act that was passed after the September 11 attacks in 2001. The act expanded government surveillance capacities, and those provisions were meant to sunset, but many are still in effect.
There’s no simple way to address these concerns. Policymakers want their COVID response to be as effective as possible, but they don’t want to open the door to abuses of private data. There are good guidelines and frameworks that exist, but many are being stress-tested now for the first time.
We’re talking about AI and machine learning, but you keep coming back to the point that this is a human endeavor.
The algorithms are sort of the shiny object, and they receive a lot of attention. But when it comes to actually implementing social protections, going the last mile to put money in the hands of people who need it, the algorithms are just one small link in a much larger chain of humanitarian assistance. Most of the other links are human. Algorithms can help surface relevant information, but humans must decide what to do with it.