New Research Combats Burgeoning Threat of Deepfake Audio
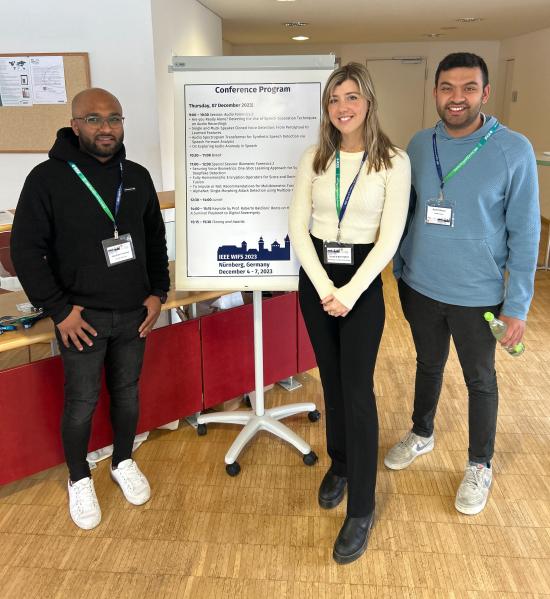
With every passing day, it seems like it is getting harder to trust what you see — and hear — on the internet. Deepfakes and doctored audio have become easier to create with the press of a button. New research by three School of Information students and alums will make it easy to determine the authenticity of an audio clip.
Romit Barua, Gautham Koorma, and Sarah Barrington (all MIMS ’23) first presented their research on voice cloning as their final project for the Master of Information Management and Systems degree program. Barrington is now a Ph.D. student at the I School. Working with Professor Hany Farid, the team looked into different techniques for differentiating a real from a cloned voice designed to impersonate a specific person.
“The team has done important work in laying out a range of ideas for detecting the new threat of deepfake audio.”
— Hany Farid
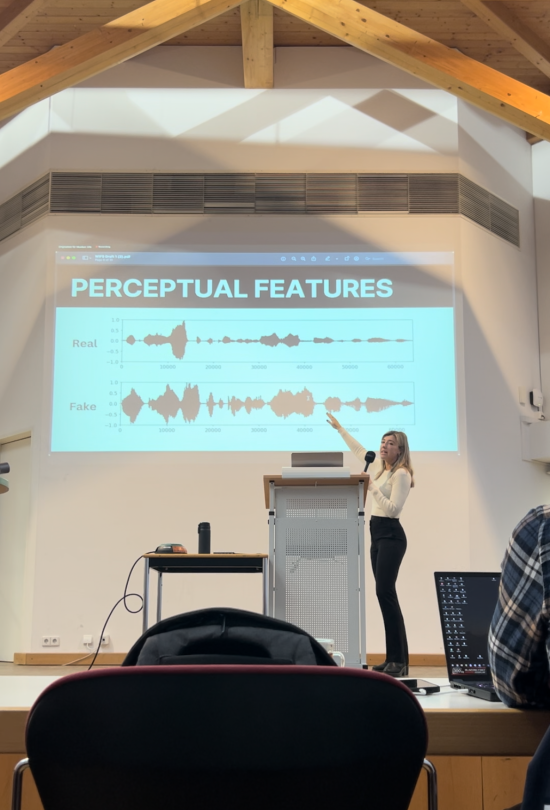
When this team first approached me in early Spring of 2022, I told them not to worry about deepfake audio because voice cloning was just not very good and it would be a while before we had to worry about it. I was wrong, and a few months later, AI-powered voice cloning was shockingly good, revealing how fast this technology evolves,” said Professor Farid. “The team has done important work in laying out a range of ideas for detecting the new threat of deepfake audio.”
To begin, the team first analyzed audio samples of real and fake voices by looking at perceptual features or patterns that can be visually identified. Through this lens, they focused on looking at audio waves and noticed that real human voices often had more pauses and varied in volume throughout the clip. This is because people have the tendency to use filler words and may move around and away from the microphone while recording. By analyzing these features, the team was able to pinpoint pauses and amplitude (consistency and variation in voice) as key factors to look for when trying to determine a voice’s authenticity. However, they also found that this method — while easy to understand — may yield less accurate results.
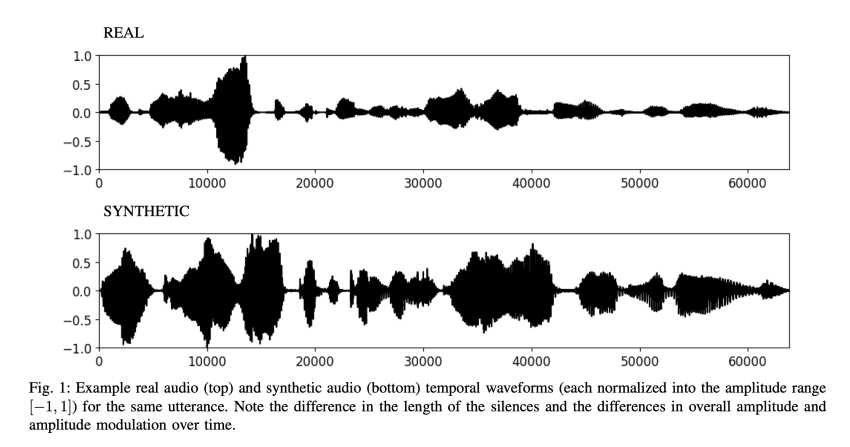
The team then took a more detailed approach, looking at general spectral features using an ‘off-the-shelf’ audio wave analysis package. The program extracts over six thousand features — including summary statistics (mean, standard deviation, etc.), regression coefficients, and more — before reducing the number to the twenty most important ones. By analyzing these extracted features and comparing them to other audio clips, Barrington, Barua, and Koorma utilized these features to create a more accurate method.
However, their most accurate results occurred with their learned features, which involves training a deep-learning model. To do so, the team feeds the raw audio to the model, from which it processes and extracts multi dimensional representations — called embeddings. Once generated, the model uses these embeddings to distinguish real and synthetic audio. This method has consistently outperformed the previous two techniques on accuracy and has recorded as little as 0% error in lab settings. Despite the high accuracy rate, the team has noted that this method could be difficult to understand without proper context.
The team believes that this research may address growing concerns about using voice cloning and deepfakes for nefarious purposes. “Voice cloning is one of the first instances where we’re witnessing deepfakes with real-world utility, whether that's to bypass a bank’s biometric verification or to call a family member asking for money,” Barrington explained. “No longer are only world leaders and celebrities at risk, but everyday people as well. This work represents a significant step in developing and evaluating detection systems in a manner that is robust and scalable for the general public.”
“This work represents a significant step in developing and evaluating detection systems in a manner that is robust and scalable for the general public.”
— Sarah Barrington
After publishing this research online, Barrington, Barua, and Koorma were invited to present their findings at various prestigious conferences, such as the Nobel Prize Summit and the IEEE WIFS (Workshop in Information Forensics and Security) conference in Nuremberg, Germany.
“WIFS provided an excellent forum for engaging with researchers in digital forensics, deepening our knowledge of state-of-the-art forensic techniques through detailed presentations and enriching peer discussions,” said Koorma.
“[It also] awarded us a great opportunity to see the research of leaders in our field as well as find common ground for future collaboration in the area of deepfake detection,” Barua added.
As society grapples with the implications of deepfakes affecting not only world leaders and celebrities but everyday individuals, this research offers a robust and scalable approach to safeguarding the general public. Delving into perceptual features, spectral analysis, and leveraging advanced deep learning models has yielded promising results, and the team’s work stands as a crucial step towards restoring trust in audio content online and mitigating the risks posed by advancing technology.