Meet Berkeley’s new data science leader
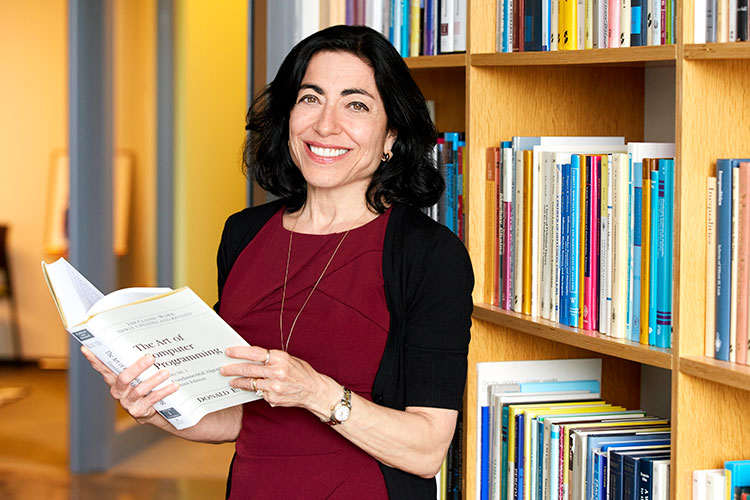
As a Microsoft technical fellow, Jennifer Tour Chayes has made a name for herself as an expert in the field of network science and a leader of multidisciplinary labs that bring data science tools to bear on a wide range of problems. In January, she will leave her current position at Microsoft to become UC Berkeley’s first associate provost for the Division of Data Science and Information and Dean of the School of Information.
Berkeley News spoke with Chayes about her research, her passion for encouraging people from underrepresented groups to pursue careers in STEM fields and her hopes for the new Division of Data Science and Information at Berkeley.
Berkeley News: At Microsoft, you are known for your work in network science. What is network science, and what fascinates you about it?
Networks explain a lot of what appears to be mysterious in the world. Often, you see only a certain signal, but you don’t understand the underlying network that produced that signal. For example, in a social network, you don’t know exactly who is connected with whom, or the strength of one person’s influence on another, but you do see some output of this network: Certain things become popular, or there are polarizations of groups. But if you could understand that network, and how to think about the structure of the network from the output, you might be able to do intervention.
In recent years, you have dedicated much of your time to studying ethics in data science. What are some of the ethical issues currently facing the field, and what is being done to address them?
Even if you try to remove protected attributes in a data set, like gender or race, a blind application of machine learning will pick up proxies, or substitutes, for those characteristics and increase bias. For example, zip code is often a proxy for race or socioeconomic level. So, though you may be attempting to exclude race from consideration from an algorithm, the outcome or the prediction will still be very biased.
These issues come up in determining loans, in determining bail, in deciding to whom a company should offer a job or an interview. So, it is very important to identify biases inherent in the data set and design algorithms that mitigate bias in those data sets. It’s possible to do that, but you really need to be very intentional about it from the beginning.
In addition to your research at Microsoft, you have also been an outspoken advocate of diversity and inclusion in STEM. What motivated you to become active in those efforts?
You know, it’s funny, I am one of those strange people who, when something scares me, I rush toward it. In the four years that I was a graduate student at Princeton, I was the first woman to get a Ph.D. in either math or physics. I would go to conferences where there would be a hundred people, and I was the only woman. And I thought that there was no gender discrimination, I was sure of it. And it was only when I became a professor at UCLA, and I saw immensely talented undergraduate women making decisions not to go on in STEM fields, that I realized that other women did not react like I react. When the environment was hostile or unwelcoming or demeaning, they did not charge toward it, they ran away from it, because they were talented and they could do something else. And so, it was at that point, when I saw the effect on phenomenal students, that I began to realize, yes, there is a problem. And then I began to get involved.
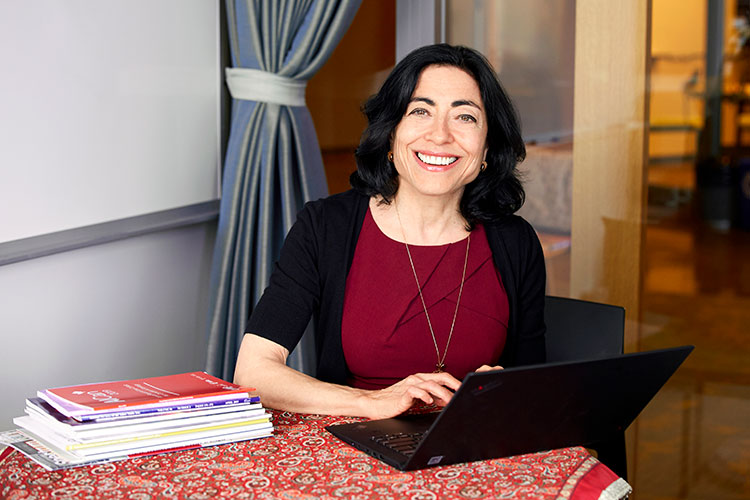
You took a non-traditional path in your education, dropping out of high school before enrolling in Wesleyan and graduating first in your class in biology and physics. What advice do you have for others who are interested in pursuing a career in data science, but think it might be too late?
One wonderful thing about not being perfect early in your life, being far from perfect, is that you become less risk-averse. And I think, to be a great scientist, to be a great contributor to any field, you need to not be too risk averse. You need to try things that are hard and at which you may fail. So, actually I think having a non-traditional background ultimately really pays off. You have to work hard, you have to overcome whatever impediments have been created, but once you get there, you are actually in much better shape, because you know you can recover.
I read that, at one point, you wanted to become an artist. Do you still appreciate art?
Yes, I had wanted to be an artist when I was very young. My mother was an artist, and I won awards in art when I was young. And, interestingly, I feel that, in my career, I’ve been an artist — just in a different medium. You know, mathematics is beautiful, it really is. When I see a beautiful proof, it sets off the same endorphins as if I am seeing a wonderful piece of art, or hearing amazing music. So, I believe that many of your faculty are actually artists in their own way, using different media.
What projects are you excited to tackle once you start at Berkeley?
One thing that I am very excited about is to be a part of the new data science major and minors in partnership with all the other wonderful schools, colleges, at Berkeley. And I hope that we might even be able to create a class that almost every undergraduate takes, which allows them to be able to understand the results of data analysis in a critical and confident way. I also hope to create professional master’s programs — not just in data science, but also in partnership with other schools.
So, on the educational front, I’m very excited about that. But I’m also coming to Berkeley for the research. I’ve been building multidisciplinary groups my entire career, but I’ve never been able to do it at the scale that we will be able to do it together at Berkeley. I would love to seed research at Berkeley in certain areas that bring people across the campus together.