Berkeley Lab-led institute to help solve data-intensive science challenges
As scientists around the world address some of society’s biggest challenges, they increasingly rely on tools ranging from powerful supercomputers to one-of-a-kind experimental facilities to dedicated high-bandwidth research networks. But whether they are investigating cleaner sources of energy, studying how to treat diseases, improve energy efficiency, understand climate change or address environmental issues, the scientists all face a common problem: massive amounts of data which must be stored, shared, analyzed and understood. And the amount of data continues to grow – scientists who already are falling behind are in danger of being engulfed by massive datasets.
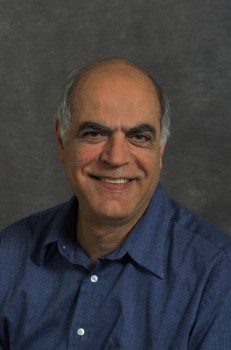
Today Energy Secretary Steven Chu announced a $25 million five-year initiative to help scientists better extract insights from today’s increasingly massive research datasets, the Scalable Data Management, Analysis, and Visualization (SDAV) Institute. SDAV will be funded through DOE’s Scientific Discovery through Advanced Computing (SciDAC) program and led by Arie Shoshani of Lawrence Berkeley National Laboratory (Berkeley Lab).
As one of the nation’s leading funders of basic scientific research, the Department of Energy Office of Science has a vested interested in helping researchers effectively manage and use these large datasets.
SDAV was formally announced March 29 as part of the Obama Administration’s “Big Data Research and Development Initiative,” which was announced this morning and takes aim at improving the nation’s ability to extract knowledge and insights from large and complex collections of digital data.
Among the other projects announced was a $10 million award to the University of California, Berkeley, as part of the National Science Foundation’s “Expeditions in Computing” program. The five-year NSF Expedition award to UC Berkeley will fund the campus’s new Algorithms, Machines and People (AMP) Expedition. AMP Expedition scientists expect to develop powerful new tools to help extract key information from Big Data. Read the UC Berkeley announcement.
SDAV is a collaboration tapping the expertise of researchers at Argonne, Lawrence Berkeley, Lawrence Livermore, Los Alamos, Oak Ridge and Sandia national laboratories and in seven universities: Georgia Tech, North Carolina State, Northwestern, Ohio State, Rutgers, the University of California at Davis and the University of Utah. Kitware, a company that develops and supports specialized visualization software, is also a partner in the project. SDAV will be funded at $5 million a year for five years. The project builds on technologies built by previous SciDAC projects in the areas of scientific data management and scientific visualization and analytics, and applied to various application domains.
As supercomputers have become ever more powerful – now capable of performing quadrillions of calculations per second – they allow researchers to conduct detailed simulations of scientific problems at an unprecedented level of detail.
For example, scientists developing a new generation of particle accelerators with applications ranging from nuclear medicine to power generation can simulate fields with millions of moving particles. But that makes it difficult to pull out the most interesting information, such as just the particles that are energetic. In the past, it could take hours to sift through the data, but FastBit, an innovative method for indexing data by characteristic features, allows researchers to perform the task in just seconds, dramatically increasing scientific productivity. At the same time, by reducing the amount of data being visualized, they will be able to see phenomena they would otherwise be unable to see.
The next step to be tackled under SDAV is to develop a way to interact with the data as it is being created in a simulation. This technique would allow researchers to monitor and steer the simulation, adjusting or even stopping it if there is a problem. Because such simulations can run for hours or days on thousands of supercomputer processors, such a capability would help researchers make the most efficient use of these high-demand computing cycles. Similarly, such tools will allow scientists to analyze and visualize data as it is being generated and could help them summarize and reduce the amount of data to a manageable level, resulting in datasets with only the most valuable aspects of the simulated experiment.
This capability will also benefit scientists using large scale experimental facilities, such as DOE’s Advanced Light Source where scientists use powerful X-ray beams to study materials. Previously, data was collected at one frame per second, but is now up to 100 frames per second. But the proposed Next Generation Light Source will pour out data at 1,000,000 frames per second. Again, having tools to manage the data as it is being generated is critical as the results of one experiment are often used to guide the next one. Scientists don’t’ want to have to wait six months just to sort out the science from the data. Awaiting discovery may be critical insight into the cause and treatment of diseases or the development of innovative materials for industry.
Once they have their data, scientists also need tools to efficiently explore the information. In some cases, they know what they are looking for. In combustion simulations, for instance, the flame front is characterized by high temperatures, chemicals to be burned, etc. In hurricane simulations, temperatures, wind velocity are key factors for turbulent behavior. Applications can be developed to look for development of such patterns and focus the computing power on those areas.
A bigger challenge is when the scientists aren’t sure what they are looking for. One example is in fusion energy reactors in which plasmas will be heated to 100 million degrees Celsius, then squeezed by powerful magnetic fields to fuse the atoms and release more energy in the process. Detailed simulations are critical to designing such reactors. The performance of the reactor depends on the ability to avoid disruptive instability occurring in the edge region, which can push the plasma out to the walls of the reactor, causing it to shut down. Mining the data to find the patterns which determine whether the simulation will proceed successfully can help researchers catch problems early and modify the parameters to eliminate these patterns.
This ability to monitor the workflow of an experiment or simulation will then be incorporated into what’s known as a dashboard, a desktop computer interface that allows users to control their project, just as an automobile dashboard gives drivers the information they need to reach their destinations.
SDAV is based on the premise that all computational scientists are facing data management problems, even if it isn’t apparent. For example, having too much data for a computer simulation can dramatically slow a supercomputer’s performance as it moves data in and out of processors. This not only wastes time, but also the power needed to run the system. By developing methods to manage, organize, analyze and visualize data, SDAV aims to greatly improve the productivity of scientists.
SDAV is also addressing the expected changes in supercomputing architectures, in which hundreds of thousands or even millions of processor cores will be packed into powerful systems. With so many processors, the ability to minimize data movement in and out of cores will be even more critical to efficient computing. Complicating the situation will be the increasing deployment of supercomputers using different types of processors, or hybrid architectures.
In the end, SDAV aims to deliver end-to-end solutions, from managing large datasets as they are being generated to creating new algorithms for analyzing the data on emerging architectures. Finally, new approaches to visualizing the scientific results will be developed and deployed based on other DOE visualization applications such as ParaView and VisIt. Kitware, the company which supports the Visualization Toolkit that underlies ParaView and VisIt, will participate in SDAV to adapt the software to the hybrid architectures.
Shoshani, who is the director of SDAV, and recently co-edited the book Scientific Data Management: Challenges, Technology, and Deployment, calls the project “the best of everything being done in DOE and the universities in these domains. This team is the cream of the crop.”