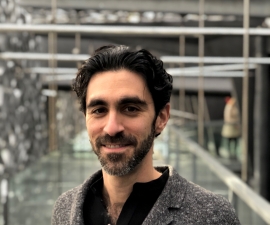
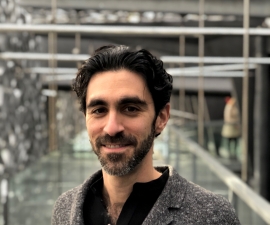
Research Expertise and Interest
machine learning, and medicine, health policy
Research Description
Ziad Obermeyer works at the intersection of machine learning and health. His research focuses on how machine learning can help doctors make better decisions (like whom to test for heart attack), and help researchers make new discoveries—by ‘seeing’ the world the way algorithms do (like finding new causes of pain that doctors miss, or linking individual body temperature set points to health outcomes). He has also shown how widely-used algorithms affecting millions of patients automate and scale up racial bias. That work has impacted how many organizations build and use algorithms, and how lawmakers and regulators hold AI accountable. That work has impacted how many organizations build and use algorithms, and how lawmakers and regulators hold AI accountable, culminating in testimony before the Senate Finance Committee in 2024.
He is one of TIME Magazine's 100 most influential people in AI, a Chan–Zuckerberg Biohub Investigator, a Research Associate at the National Bureau of Economic Research, and was named an Emerging Leader by the National Academy of Medicine. Previously, he was Assistant Professor at Harvard Medical School, and continues to practice emergency medicine in underserved communities.
In the News
New Center Aims To Create Healthcare Innovation Research-To-Impact Pipeline
Chan Zuckerberg Biohub Awards $21 Million to 21 Berkeley Researchers
Understanding and seeking equity amid COVID-19
Widely used health care prediction algorithm biased against black people
Featured in the Media
An algorithm widely used by health insurers to make critical care decisions reflects strong racial biases, a team of scientists led by acting associate public health professor Ziad Obermeyer MD has found, and that has led to poorer outcomes for black patients, compared to white patients. "We shouldn't be blaming the algorithm," Dr. Obermeyer says. "We should be blaming ourselves, because the algorithm is just learning from the data we give it." Setting out to fix the problem, Dr. Obermeyer's team developed an alternative model that reduced the bias by 84%, and shared it with the algorithm's manufacturer. For more on this, see our press release at Berkeley News. Stories on this topic have appeared in dozens of sources around the world, including KQED Radio's Forum (link to audio), Managed Healthcare Executive, Health IT Analytics, News-Medical, Market Screener, and Mic.