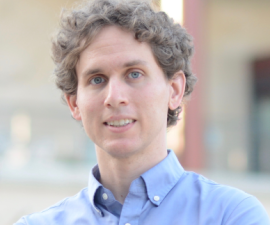
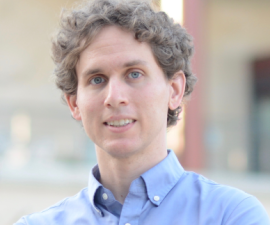
Research Expertise and Interest
artificial intelligence, machine learning
Research Description
Jacob Steinhardt's goal is to make the conceptual advances necessary for machine learning systems to be reliable and aligned with human values. This includes the following directions:
- Robustness: How can we build models robust to distributional shift, to adversaries, to model mis-specification, and to approximations imposed by computational constraints? What is the right way to evaluate such models?
- Reward specification and reward hacking: Human values are too complex to be specified by hand. How can we infer complex value functions from data? How should an agent make decisions when its value function is approximate due to noise in the data or inadequacies in the model? How can we prevent reward hacking--degenerate policies that exploit differences between the inferred and true reward?
- Scalable alignment: Modern ML systems are often too large, and deployed too broadly, for any single person to reason about in detail, posing challenges to both design and monitoring. How can we design ML systems that conform to interpretable abstractions? How do we enable meaningful human oversight at training and deployment time despite the large scale? How will these large-scale systems affect societal equilibria?
These challenges require rethinking both the theoretical and empirical paradigms of ML. Theories of statistical generalization do not account for the extreme types of generalization considered above, and decision theory does not account for cases where the reward function is only approximate. Meanwhile, measuring empirical test accuracy on a fixed distribution is insufficient to analyze phenomena such as robustness to distributional shift.