Computer Vision to Protect Patients — and Budgets
Alzheimer’s disease not only robs people of memories and cognitive skills, but over time, it increases their vulnerability to falling down, and suffering a head injury.
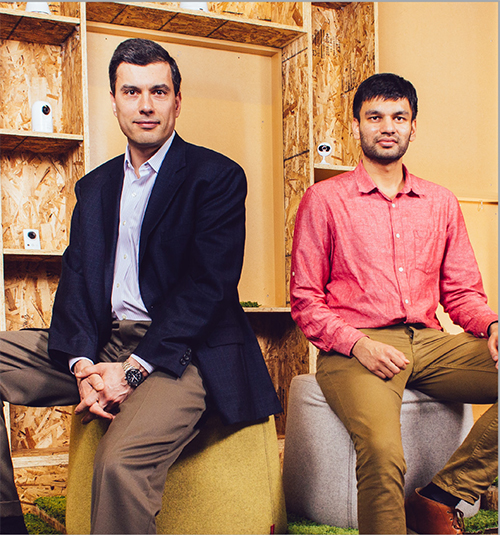
State regulations require an MRI of the head any time a patient suffers an unwitnessed fall, and about a fourth of all Alzheimer’s-related hospital visits are triggered by a fall.
With five million Americans currently living with Alzheimer’s, the task of preventing, tracking and treating fall-related injuries has become daunting and costly, with more than a $5 billion annual cost to medicare. And the number of people with Alzheimer’s is expected to double in the next 15 years.
Memory care centers make every effort to detect and prevent falls, but monitoring patients around the clock or watching video of a patient’s full nighttime activity is labor intensive and expensive. Another strategy — asking patients to wear pendants that detect falls — becomes impractical when forgetful patients fail to wear the devices.
A few years ago, UC Berkeley computer science PhD student Pulkit Agrawal turned to research applying computer vision to improve medical care of people with Alzheimer’s.
“There are no effective drugs yet to treat Alzheimer’s,” he says. “Until we have them, we have to help patients where they are. Developing computer vision systems to detect falls and fall vulnerability seemed like a good way to improve healthcare for a growing patient population.”
A system capable of detecting falls by autonomously monitoring patients could help therapists by only sending them video clips when a patient stumbles or falls, he says.
Agrawal partnered with Alexandre Bayen, professor of electrical engineering and computer sciences, an expert in mobile sensing to design and eventually market a reliable, inexpensive computer vision technology to improve detection and risk of falls.
Along with team members, Julien Jacquemot, visiting scholar at UC Berkeley, and PhD student George Netscher, they saw the potential of passive vision-based sensing technology to overcome the limitations of the previous approaches.
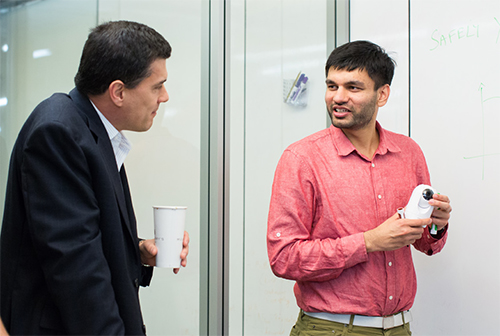
With support from the Signatures Innovation Fellows program, they are already developing an automated online detection system for use in large networks of memory care facilities.
Agrawal employed a new artificial intelligence method known as deep learning to train a computer to identify falls and distinguish them from unsteady movements and other patient behavior.
The deep learning algorithms are taught to detect falls by the process of trial and error. In contrast to earlier computer vision methods, deep learning methods are known for steadily improving their performance as they are fed more data. This means that this fall detection system only gets better with time.
The current system provides live video streaming of patients and alerts staff when it detects a fall.
To assure maximum patient privacy, only videos of falls are saved for review, and non-fall videos are automatically deleted within a minute. Therapists and physicians have 24-hour-a-day access to the videos via a secure website.
“We think we’re developing a reliable system that can prevent patient injury and quickly respond to falls, while keeping costs down at a time when this patient population is only growing,” says graduate student Netscher.
In an initial pilot project, occupational therapists were able to identify typical causes of falls, leading to an 80 percent reduction in falls over three months in a subset of patients in a single facility.
The team has expanded the effort, working with clinicians in memory care facility networks in California. More pilots are planned, with a goal of testing the detection system by monitoring up to 1,000 patients by end of 2019.
The team also anticipates a broader potential for the technology, such as bed sores prevention and unsafe wandering.
“We want to improve the way memory care facilities operate by empowering caregivers with more options than they currently have,” Bayen says.
____________________________
The Signatures Innovation Fellows Program supports innovative research by UC Berkeley faculty and researchers in the data science and software areas with a special focus on projects that hold commercial promise.
For more information, see http://vcresearch.berkeley.edu/signatures/about.