When Data Science Meets Medicine
Bin Yu, a 2022 Fellow with the UC Noyce Initiative, is applying cutting edge data science techniques to pressing issues in health and medicine
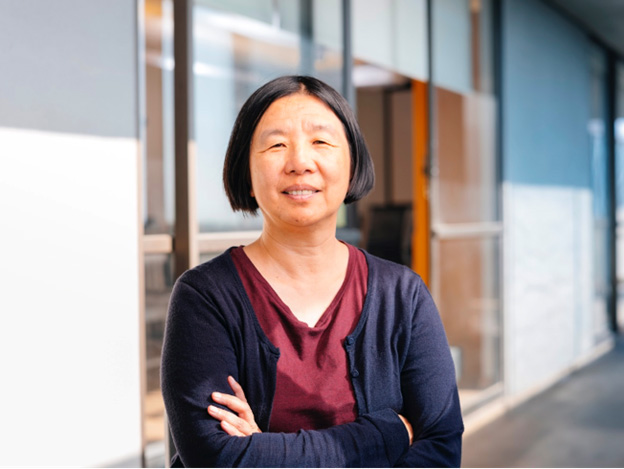
As a child, Bin Yu never dreamed she’d go to college. She grew up in China during the Cultural Revolution, when nearly all of the country’s institutions of higher learning were closed. But in third grade, a cousin gave Yu a math book. She fell in love with the structured way of thinking and the concrete answers found in the textbook.
“Math really became a refuge from all the turmoil around me,” says Yu, now a professor of statistics, of electrical engineering and computer sciences, and of computational biology at UC Berkeley. Over time, this refuge led her down an academic path—first attending college in China when schools began to reopen, and then winning a fellowship to UC Berkeley.
Today, Yu immerses herself in statistics, data science, and machine learning not as an escape mechanism but a way to solve large-scale problems, many of them in the healthcare arena. Early in the COVID-19 pandemic, for instance, she and her research team spearheaded a new way to predict which hospitals would need personal protective equipment (PPE). Their modeling helped guide one non-profit organization’s shipments of more than 340,000 pieces of PPE to the most at-need medical facilities.
Most recently, as a 2022 Fellow with the UC Noyce Initiative, Yu is using her data analysis approach to help physicians decide whether young children in the emergency room should get CT scans, based on live feeds of information about the children’s symptoms, vital signs, and test results.
Driven by Social Responsibility
After becoming immersed in her cousin’s math book, Yu wanted to keep studying math, but wasn’t sure what that path would look like. A middle school teacher and mentor encouraged Yu to apply to study math in Peking University, and seeded the idea of graduate school in the United States in Yu’s mind.
“I knew that I wanted to see the world, and so I really liked that idea,” says Yu, “It encouraged me to keep studying English beyond the basic two years required by my college, so that one day I could come to the U.S.”
At the end of college, Yu was the top ranked student in her major in the graduate school entrance exam, but didn’t get selected by the math department to work with the math professor that she wanted — she thought then, and thinks now, her gender may have played a role. Frustrated, she switched from math to statistics. The decision turned out to have lasting effects on her career; not only did she become enamored with statistics, but she won a last-minute fellowship to study in the U.S. She would spend a year in Nankai University studying English and then begin a PhD program in statistics at Berkeley.
While Yu enjoyed the pure elegance of math, she came to see how statistics could tackle the world’s problems in new ways.
“Over the years, a lot of people in my family gave up a lot to do the right thing,” says Yu. “It engrained in me this sense of social responsibility that has stayed with me throughout my career. I think statistics, more than math alone would have, has let me be more useful in this way and contribute to society directly.”
Taking on Medicine
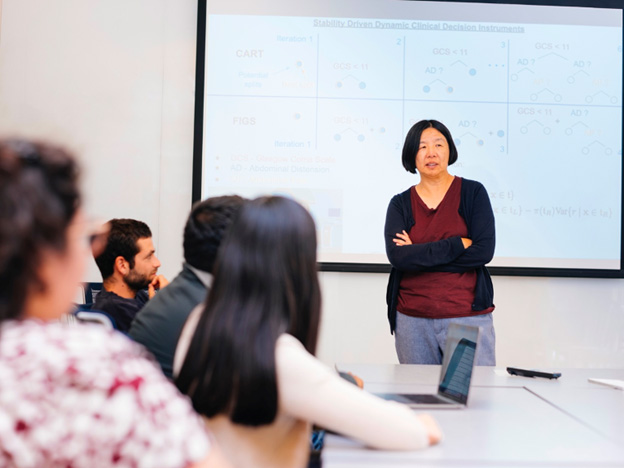
Over the past three decades at Berkeley, Yu has combined statistics, data science, and machine learning to study basic biological questions in fields like neuroscience and genetics. She has also developed a new framework called veridical (truthful) data science for other data scientists to ensure that their data analysis—and conclusions—are transparent and accurate. Much of this revolves around how scientists “clean” their datasets, picking and choosing which pieces of data to include in a study.
“Two people can take the same set of data and come to completely different conclusions because of the way they’re cleaning the data,” says Yu. “We want to make sure data conclusions can be replicated and trustworthy.”
In 2014, Yu had a major surgery and spent time in the hospital. She began to see that her fair, transparent, unbiased way of handling data could be especially useful in analyzing health data. She began to shift her lab’s focus toward medicine and healthcare.
“Medicine is an area that needs very rigorous thinking and careful data analysis,” says Yu. “I feel like it’s my professional responsibility to make a difference in this field.”
With the UC Noyce Initiative funds, Yu is lending her expertise to a trade-off that has stumped emergency room doctors for decades: CT scans expose children to a potentially dangerous amount of radiation, so are used sparingly; but avoiding the scans too often can mean missing internal bleeding. Yu and her students, working with Dr. Aaron Kornblith at UCSF, are developing algorithms that periodically scan children’s medical records while they are in the ER — including real-time data on hospitalized patients— for clues about whether the radiation exposure of a CT scan is worth the risk.
“In the longer run, I think the approach we’re developing could actually be used in all sorts of medical contexts, including chronic disease diagnosis,” says Yu. “My goal isn’t just to tackle one small problem at a time; it’s to bring all sorts of skills and people together to create entirely new frameworks for analyzing data in order to arrive at trustworthy conclusions such as clinical decision rules for sending kids to CT scan in ER.”