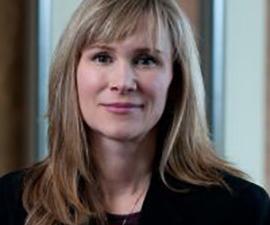
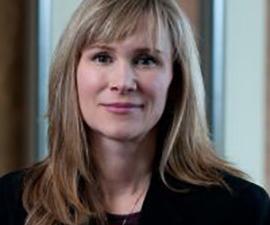
Research Expertise and Interest
psychology, mental health, criminal justice, risk assessment, intervention
Research Description
Jennifer Skeem is a psychologist who writes and teaches about the intersection between behavioral science and criminal justice. Her research is designed to inform efforts to prevent violence, improve decision-making about people involved in the justice system, and achieve effective and equitable justice reform. Current projects include testing innovative correctional services for people with mental illness, identifying environmental factors that promote violence within institutions, and promoting prosocial behavior among juveniles at risk. Much of Skeem’s current work addresses a surge of interest in the use of risk assessment to inform criminal sentencing—including how this practice may affect racial and economic disparities in imprisonment.
Skeem has authored over 150 articles and edited 2 books—including Applying Social Science to Reduce Violent Offending. She is past President of the American Psychology-Law Society and member of the. MacArthur Foundation Research Network on Mandated Community Treatment. Skeem has delivered congressional briefings on her work and consults with local and federal agencies on issues related to prevention of (mass) violence, community corrections, and sentencing and prison reform.