Seeking Data Wisdom
Landing a spacecraft on a hurtling comet or cloning genes of extinct animals. Science and engineering have a way of turning what seems like fantasy into solid reality. Now add the prospect of someone reading your mind.
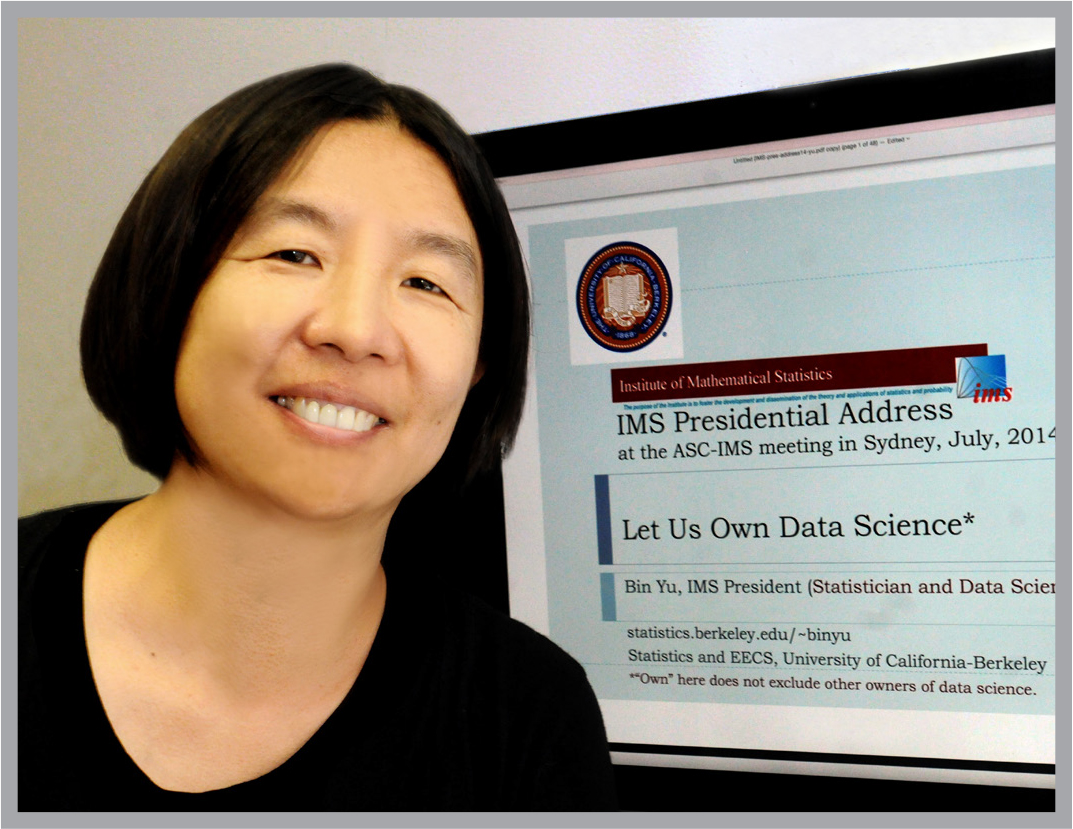
They’re not there yet, but in 2011, Berkeley scientists startled colleagues and triggered imaginations by divining the rough outlines of what experimental subjects were seeing in movie clips. They accomplished this using a type of MRI to detect indirectly neuron firing at precise locations in the brain’s visual processing area.
The achievement coupled basic science, intense computation and complex statistical analysis. Matching thousands of individual movie frames with the MRI signal detected at the instant the image was seen, the researchers were able to produce fairly reliable outlines of what their subjects were seeing from the specific MRI signature.
The experiments used functional MRI, or fMRI, to measure blood flow in precise areas of the brain’s visual processing region. The fMRI readings served as a proxy for neuron firing.
The research was based on a widely held theory that the shapes we see are the product of different patterns of neuron activity in precise areas of the brain’s visual processing center, located in the cortex. Different patterns: different perceptions. Neuroscientists Jack Gallant and post-doc Shinji Nishimoto led the brain imaging. Statistician and data scientist Bin Yu and her former grad student Yuval Benjamini joined Nishimoto and the Gallant Lab to design the statistical algorithms.
Yu and her team had to analyze a torrent of fMRI data to identify from thousands of movie clips the 100 frames that most likely matched a given voxel activity pattern. They then “averaged” these shapes to yield the outline of what the subjects were seeing.
“In computational neuroscience, it is important to gauge how much variation there is in the signals — in this case, how much of this variation is due to the movies and how much is due to “noise,” Yu says. “This is absolutely essential to determine how well the frames of a movie clip are encoded in the brain.”
Yu is now leading a project in collaboration with the Gallant Lab to apply statistical machine learning analysis to determine how a neuron in a key visual area of the brain, called V4, responds to contours, and to the ability of the brain’s visual system to differentiate foreground and background in an image. These capabilities add crucial detail to what we see.
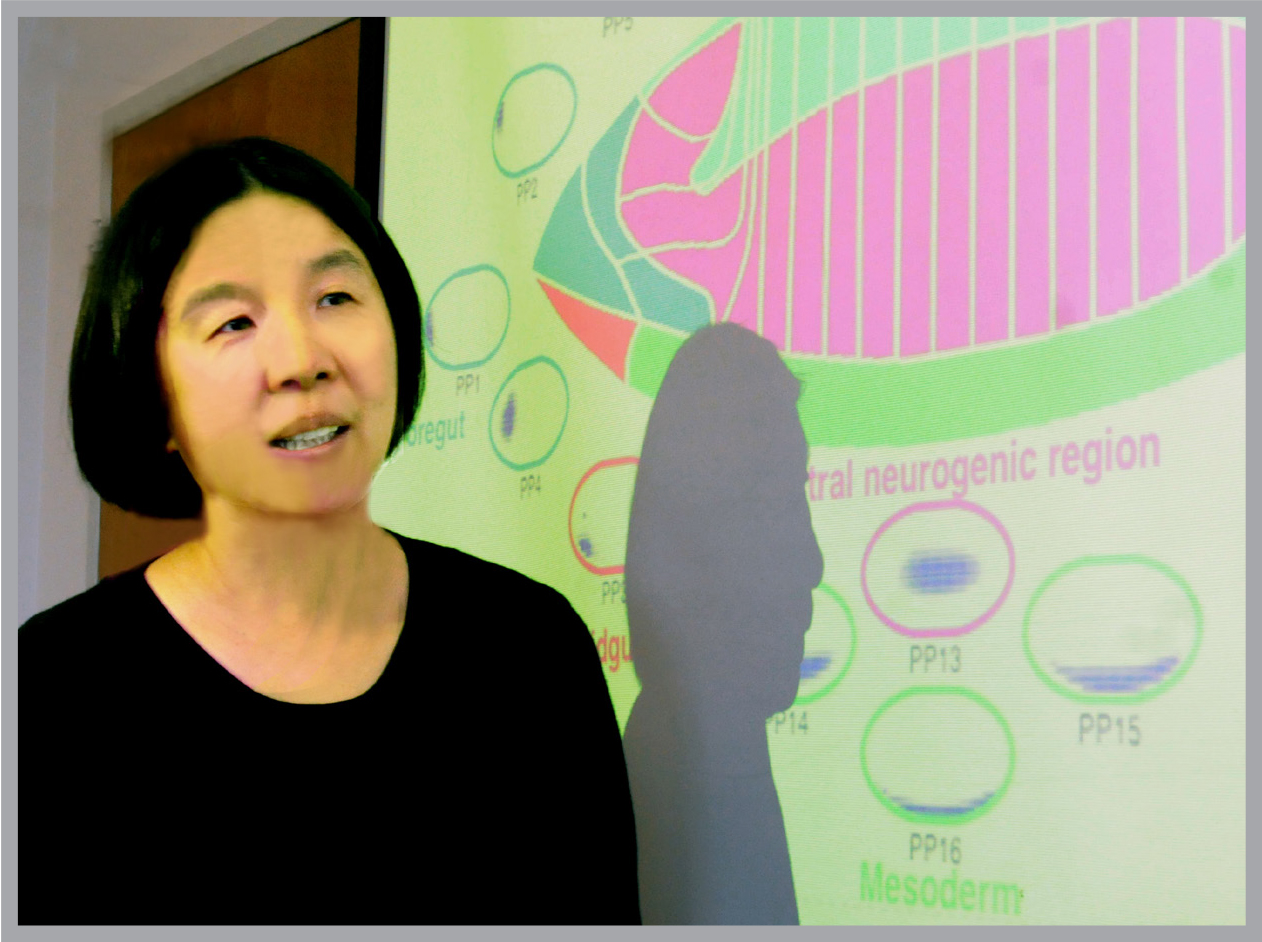
She thinks that only a powerful interlocking of science, computation and statistics could have led to the Berkeley “mind-reading” success. This synergy, she says, is an underappreciated aspect of data-intensive research today. In her view, such studies can only yield solid results if they employ “data wisdom”* — her rebranding of the best of applied statistics.
Like a powerful telescope or precision gene microarray, statistical analysis is a tool – what Yu calls a “soft lens” — essential for scientists in all data-intensive disciplines to make discoveries and assure the significance of their results. She collaborates with colleagues in neuroscience, developmental biology and political science to strengthen research design and to assess reliability of results.
Using an approach inspired by the neuroscience research, Yu, graduate student Siqi Wu and others are developing statistical tools to explore which genes interact in the developing Drosophila, or fruitfly, embryo. Gene-gene interactions are profoundly important for the formation of organs at the right time and in the right place in organisms, from the fly to humans. The research is co-led by LBNL biologist Erwin Frise as part of the Berkeley Drosophila Genome Project, in collaboration with his LBNL colleague Sue Celniker, and with computer scientist Wei Xu of Tsing Hua University.
A better understanding of the genetic underpinnings of development may ultimately aid treatment of developmental disorders and cancer, Yu says. But the effort poses major hurdles.
“There is now an abundance of data with spatial information,” she says. “We can study organisms in amazing detail, but the data is huge and complex. Under our “soft-lens,” beautiful structures hidden in data reveal themselves, such as regions in the embryo that are destined to become organs.
“It is challenging and exciting to extract knowledge from these data. It takes a real team effort — biologists, statisticians, computer scientists and a focus on data wisdom.”